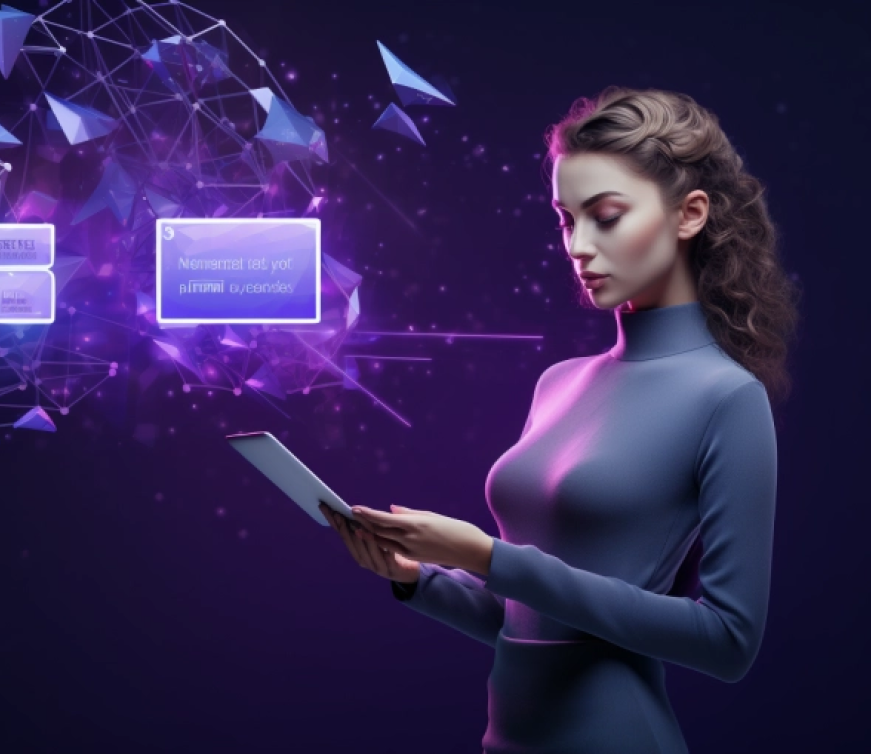
Neural Networks and Deep Learning
Andrew Ng
Instructed by AI pioneer Andrew Ng, Generative AI for Everyone offers his unique perspective on empowering you and your work with generative AI. Andrew will guide you through how generative AI works and what it can (and can’t) do. It includes hands-on exercises where you’ll learn to use generative AI to help in day-to-day work and receive tips on effective prompt engineering, as well as learning how to go beyond prompting for more advanced uses of AI.
View MoreAbout this Course
In the first course of the Deep Learning Specialization, you will study the foundational concept of neural networks and deep learning.
By the end, you will be familiar with the significant technological trends driving the rise of deep learning; build, train, and apply fully connected deep neural networks; implement efficient (vectorized) neural networks; identify key parameters in a neural network’s architecture; and apply deep learning to your own applications.
The Deep Learning Specialization is our foundational program that will help you understand the capabilities, challenges, and consequences of deep learning and prepare you to participate in the development of leading-edge AI technology. It provides a pathway for you to gain the knowledge and skills to apply machine learning to your work, level up your technical career, and take the definitive step in the world of AI.
Read More- Instructor(s): Andrew Ng
Browse Lesson Plan
Introduction to Deep Learning
- Module
- •
- Module 1 - 2 Hours to complete
- Welcome Introduction
- What is Neural Network?
- Supervised Learning with Neural Networks
- Why is Deep Learning taking off?
- About this course.
- Geoffrey Hinton Interview
Neural Networks Basics
- Module
- •
- Module 2 - 7 Hours to complete
- Binary Classification
- Logistic Regression
- Logistic Regression Cost Function
- Gradient Descent
- Derivatives
- More Derivatives Examples
- Computation Graphs
- Derivative with a Computation Graph
- Logistic Regression Gradient Descent
- Gradient Descent on m Examples
- Vectorization
- More Vectorization Examples
- Vectorization Logistic Regression
- Broadcasting in Python
Shallow Neural Networks
- Module
- •
- Module 3 - 5 Hours to complete
- Neural Networks Overview
- Neural Network Representation
- Computing a Neural Network’s Output
- Vectorizing Across Multiple Examples
- Explanation for Vectorized Implementation
- Activation Functions
- Why do you need Non-Linear Activation Functions?
- Derivatives of Activation Functions
- Gradient Descent for Neural Networks
- Backpropagation Intuition (Optional)
- Random Initialization
Deep Neural Network
- Module
- •
- Module 4 - 8 Hours to complete
- Deep L-layer Neural Network
- Forward Propagation in a Deep Network
- Getting your Matrix Dimensions Right
- Why Deep Representations?
- Building Blocks of Deep Neural Networks
- Forward and Backward Propagation
- Parameters vs Hyperparameters
What does this have to do with the brain?